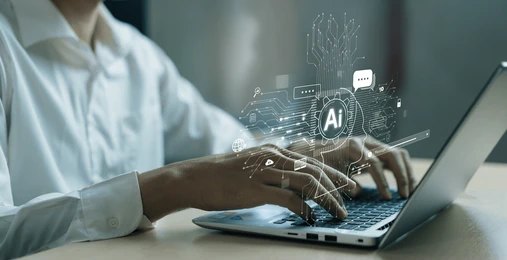
Artificial intelligence (AI) has made tremendous strides in recent years, and one of the most impressive advancements has been the development of AI-generated voices. From virtual assistants like Siri and Alexa to AI-driven voiceovers for videos and audiobooks, AI voices are now part of our daily lives. But how exactly do these AI-generated voices work? What is the science behind them?
In this blog post, we will explore the technology and techniques that power AI-generated voices, how machine learning models are trained to produce natural-sounding speech, and the future of this technology.
What Is AI Voice Generation?
AI voice generation refers to the process of creating human-like speech from text using artificial intelligence algorithms. It involves converting written text into audible speech that mimics human tones, emotions, accents, and speech patterns. This technology is powered by various techniques in machine learning, neural networks, and signal processing.
The ultimate goal of AI voice generation is to make digital voices sound as natural and expressive as human speech, opening up possibilities for applications in a wide range of industries, including entertainment, customer service, e-learning, and more.
The Role of Machine Learning and Deep Learning
1. Machine Learning Models
At the core of AI-generated voices is machine learning (ML). Machine learning is a subset of AI that enables systems to learn from data and make predictions or decisions without being explicitly programmed. In the case of AI voice generation, machine learning models are trained on large datasets of human speech to learn patterns in pronunciation, intonation, and rhythm.
For example, these models analyze audio recordings paired with corresponding text (known as a corpus) to learn how human speech sounds in different contexts. By examining millions of hours of speech data, the model can begin to generate voices that closely resemble human speech.
2. Deep Learning and Neural Networks
Deep learning is a more advanced type of machine learning that uses multi-layered neural networks to process large amounts of data. In AI voice generation, deep neural networks (DNNs) are trained to predict the next sound or word based on the input text. These models can capture the complexity of human speech, such as prosody (intonation, rhythm), accents, and emotional tones, to produce more natural-sounding voices.
WaveNet, a deep learning model developed by Google DeepMind, is one of the most advanced neural network architectures used for AI voice generation. WaveNet can synthesize human-like speech by modeling how sound waves are formed, resulting in smoother, more natural-sounding audio output.
The Process of AI Voice Generation
The process of AI voice generation typically involves several key steps, all powered by complex algorithms and models. Here’s how it works:
1. Text-to-Speech (TTS) Conversion
The first step in AI voice generation is converting written text into a machine-readable format. The AI system breaks down the input text into smaller units, such as phonemes (individual speech sounds), words, and sentences. The system then analyzes the text for pronunciation rules, grammatical structures, and punctuation to determine how it should be read aloud.
- Example: The word “read” could be pronounced differently depending on its context, and the AI model must learn to identify the correct pronunciation.
2. Phonetic and Linguistic Analysis
Once the text has been processed, the AI system performs a phonetic and linguistic analysis. This involves mapping each word to its phonetic equivalent using a dictionary of sounds. The AI model also takes into account things like stress patterns, pitch, and pacing, which vary depending on the language and context.
This step is crucial for ensuring that the speech sounds natural and not robotic. It requires the AI to learn subtle nuances, such as which syllables should be stressed in a sentence or how words should be connected smoothly.
3. Speech Synthesis
In this stage, the AI system generates the actual speech. There are two primary methods for generating synthetic speech:
- Concatenative Synthesis: This method involves piecing together pre-recorded snippets of human speech (called «units») to form complete sentences. While this approach produces more natural-sounding speech, it can be limited in its flexibility and expressiveness.
- Parametric Synthesis: This method uses mathematical models to generate speech waveforms from scratch, making it more flexible. Deep learning models like WaveNet use parametric synthesis to generate continuous speech waveforms that sound more like real human voices.
4. Voice Customization
One of the most exciting advancements in AI-generated voices is the ability to customize voices. Using deep learning techniques, voice generators can be trained to replicate specific voices, accents, or even emotional expressions.
For instance, some platforms allow users to create unique voice profiles that capture a particular speaker’s tone, pitch, and cadence. Others can simulate various emotions such as joy, sadness, or anger by adjusting the vocal tone and pacing, making the voice feel more engaging and dynamic.
Key Technologies Behind AI Voice Generation
Several key technologies work together to enable AI voice generation, each contributing to making synthetic voices sound more human-like:
1. Natural Language Processing (NLP)
Natural Language Processing (NLP) is a branch of AI that focuses on understanding and generating human language. NLP is used to analyze and interpret the text input provided by users, ensuring that the voice output reflects the correct meaning and context. It helps AI systems understand grammar, syntax, and semantics, which is essential for producing coherent and intelligible speech.
2. Text-to-Speech (TTS) Engines
TTS engines like Google Cloud Text-to-Speech, Amazon Polly, and IBM Watson Text to Speech are built using deep learning algorithms that enable highly accurate and natural speech generation. These engines are trained on vast datasets and continuously improve as they process more data.
3. Voice Cloning
Voice cloning is an exciting new feature enabled by AI voice generation technology. It allows users to create digital versions of their own voice, which can then be used for voiceovers, audiobooks, or virtual assistants. This process involves training AI models on a small sample of a person’s voice and then synthesizing that voice to generate new speech.
Example: Many voice actors now use AI tools to generate different characters’ voices or create backup recordings without needing to be present for every recording session.
The Future of AI-Generated Voices
As AI technology continues to evolve, AI-generated voices will become even more realistic and versatile. The future of this technology could see improvements such as:
- More Human-Like Voices: Advances in machine learning algorithms will allow for more lifelike voices that capture subtle human expressions, emotions, and tonal variations.
- Personalized Voices: Users will be able to create voices that match their unique characteristics, accent, and speech style.
- Real-Time Adaptation: AI voices could adapt in real-time to users’ preferences, switching tone, pitch, and speed depending on context or audience.
Conclusion
AI-generated voices are transforming how we interact with technology, providing businesses and individuals with powerful tools for communication, entertainment, and education. The science behind these voices, including machine learning, deep learning, and natural language processing, enables them to sound more natural, expressive, and versatile than ever before.
As AI continues to advance, the possibilities for AI-generated voices are boundless, opening up new avenues for creativity and innovation across various industries. Whether for customer service, content creation, or entertainment, the future of AI-generated voices is an exciting one to watch.